The XRecommend Engine composer page gives your the power to choose your model, goals, and schedule. Driven by two core recommendation models, DeepRec and QuickRec, the engine can be trained to target specific goals like Average Order Value.
Models
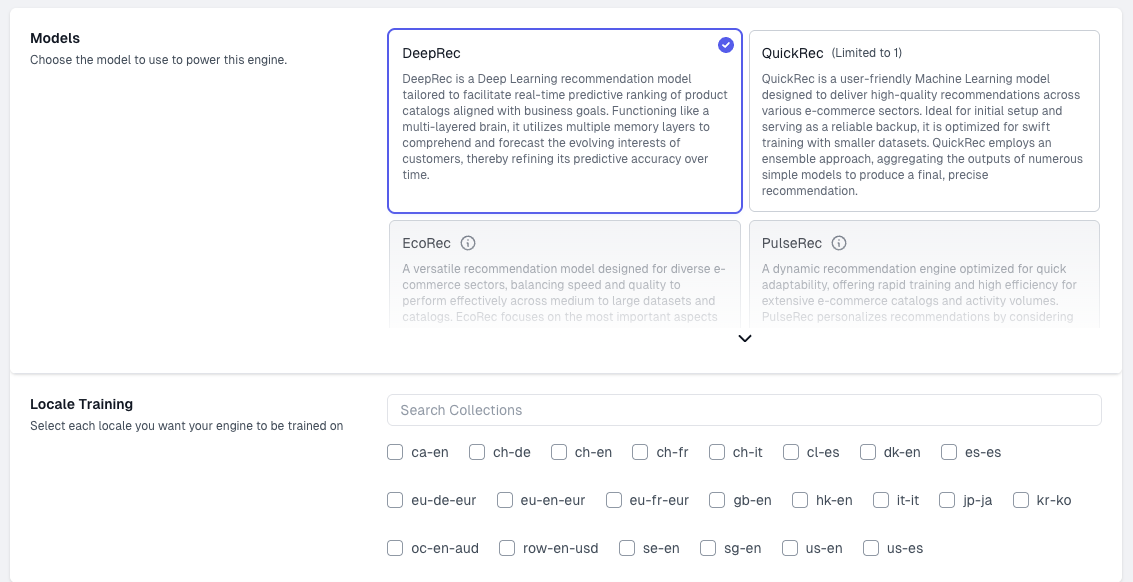
XRecommendation Engine Composer Screen - Choose Models and Locales Section [Top]
The first key setting in configuring an Engine is choosing the model.
DeepRec
A Deep Learning recommendation model tailored to facilitate real-time predictive ranking of product catalogues aligned with business goals like Conversion Rate, CTR, Margin etc.
QuickRec
A Machine Learning model designed to deliver high-quality recommendations across various ecommerce sectors. QuickRec aggregates the outputs of numerous simple models to produce one final, precise recommendation. Ideal for initial setup and as a fallback to DeepRec.
Disclaimer
Only one engine can be selected at a time. QuickRec is the automatic fallback in all instances to avoid any downtime or delays. Other engines are available for configuration but must be enabled by contacting our customer support team.
Training Goals
In order to achieve tactical business outcomes, each model can be trained on a specific Target Goal. Average Order Value (AOV), or the average amount spent by customers per order over a set period of time, is the only goal available out-of-the-box. Other Target Goals are available for configuration but must be enabled by contacting our customer support team, as some of these Target Goals require data from you outside of the standard Product Feed.
Product Prioritization (AI Boost)
Boosting a Merchandising Rule puts a weight and focus on the products the fit that rule so those products and others like them are more likely to be recommended. The DeepRec model uses your Merchandising Rule and makes inferences and connections that go far beyond manual keywording or tagging.
Product Deprioritization (AI Bury)
Burying a Merchandising Rule puts a weight and focus on the products the fit that rule so those products and others like them are less likely to be recommended. The DeepRec model uses your Merchandising Rule and makes inferences and connections that go far beyond manual keywording or tagging.
Requirement
A Merchandising Rule must be created before it is used for prioritization and deprioritization.
History
The number of days previous to today to fetch raw event data from. The higher the number of days, the more user data is captured in training, but will require tuning of other parameters to obtain optimal model accuracy
Default Look Back Period:
30 days
FAQ
What is the primary function of the DeepRec model?
DeepRec is a Deep Learning recommendation model designed for real-time predictive ranking of product catalogues based on business goals like Conversion Rate and CTR.
Can I use multiple engines at the same time?
No, only one engine can be selected at a time.
How can I access all the Training Goals available?
The only out-of-the-box training goal available is Average Order Value (AOV). Other Target Goals are available for configuration but must be enabled by contacting our customer support team, as some of these Target Goals require data from you outside of the standard Product Feed.
What is required before using product prioritization or deprioritization?
A Merchandising Rule must be created before it can be used for prioritization or deprioritization.